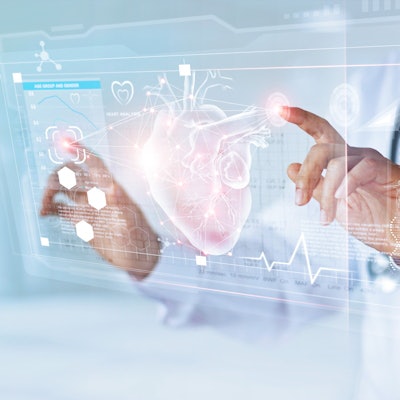
Using a convolutional neural network (CNN) with 4D flow MRI slashes interpretation time for analyzing imaging data from pediatric patients with cardiac abnormalities, according to research delivered August 12 at the International Society for Magnetic Resonance in Medicine (ISMRM) virtual conference.
The findings could be good news for both radiologists and patients, since a streamlined workflow for cardiac MRI can translate into quicker treatment planning.
"With rapid and accurate segmentation of the aorta and pulmonary arteries, 4D flow MRI data can be analyzed quickly across multiple sites," said study presenter Haben Berhane, PhD, of Lurie Children's Hospital of Chicago.
The benefits of MRI with 4D flow protocols include effective characterization and quantification of blood flow dynamics in children with congenital heart disease. But analyzing the imaging data can be challenging, "requiring extensive and time-consuming manual segmentation," Berhane noted.
Previous research has demonstrated that it's possible to automate segmentation of the thoracic aorta from 4D flow MRI scans. But Berhane's group sought to further develop these findings by including multilabel segmentation of the aorta and pulmonary arteries using multiple vendor datasets from multiple sites.
The investigators trained and validated a CNN algorithm for segmenting of the aorta and pulmonary arteries from 4D flow MRI data taken from multiple vendors and centers. The study included 67 whole-heart 4D flow MRI scans taken from pediatric patients at Lurie Children's Hospital and Children's Hospital of Colorado in Aurora. Of these, 29 showed cardiac abnormalities (such as pulmonary valve and ventricular defects, coarctations, Marfan syndrome, and tetralogy of Fallot).
Two readers used 3D phase-contrast MR angiograms generated by 4D flow data to carry out manual 3D segmentations of the aorta and pulmonary artery; these 3D data were used to train the CNN. The investigators calculated the intraclass correlation coefficients (ICC) to evaluate the CNN's performance compared with the manual segmentations, calculating net flow, peak velocity, and the ratio of total pulmonary blood flow to total systemic blood flow (Qp/Qs).
The group found that across all performance measures, the automated segments showed moderate to strong agreement with manual segments and took much less time: The CNN performed the segmentation task in 34 seconds, compared with 15 minutes for manual segmentation.
Agreement between manual and CNN aorta and pulmonary artery segmentation | |
Measure | ICC |
Aorta net flow | 0.97 |
Pulmonary | 0.99 |
Qp/Qs | 0.73 |
Using a CNN with 4D flow MRI shows promise for improving workflow -- and thus improving treatment planning for pediatric patients with cardiac abnormalities, according to the study.
"Future research will incorporate more data and build a more balanced cohort across more centers and vendors," Berhane concluded.