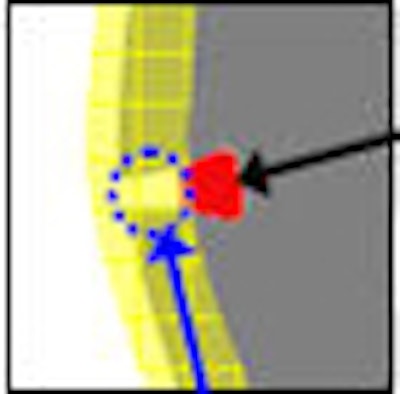
One of the thorniest challenges in computer-aided detection (CAD) is the high rate of false-positive detections, a number that generally rises in tandem with the sensitivity of the CAD algorithm. In lung CAD applications, more than one study has shown that the presence of too many false positives can mislead readers into ignoring true-positive detections in some cases and accepting false positives in others.
Yukihiro Nomura and his team from the University of Tokyo in Japan were finding too many false positives and some critical false negatives in a lung CAD application they developed utilizing "curvature-based detection and surface-feature-based false-positive reduction," Nomura explained in a presentation at the 2008 Computer Assisted Radiology and Surgery (CARS) meeting in Barcelona.
"In our preliminary study, the number of false positives at 90% sensitivity was 18.7 per case [16 cases, 73 noncalcified solid nodules]," Nomura said. "However, in our previous study some of the juxtapleural nodules also resulted in false negatives."
The system utilizes a surface-feature-based false-positive reduction with a single quadratic classifier based on Mahalanobis distance, a distance measure based on correlations between variables that permit the identification and analysis of different patterns. However, performance of the false-positive reduction scheme was limited due to the feature differences between juxtapleural and nonpleural nodules, he said.
"The objective of our study was to develop a new false-positive reduction method based on dual classifiers for juxtapleural and nonpleural nodules, and to evaluate the proposed method by comparing it with our previous method based on a single classifier," Nomura said.
The detection algorithm includes three steps: preprocessing, nodule candidate extraction, and false-positive reduction, Nomura said. First, the pulmonary volume is extracted by grayscale thresholding. Binarization is carried out to extract the region of nodules and vessels. For extraction of nodule candidates, thresholding based on the shape index value and 3D labeling are performed on the lung volume. For various sizes of nodules, multiscale regression is performed based on the eigenvalues of the Hessian Matrix to obtain the appropriate shape index values. Finally, small components under a set volume threshold are removed and shape index-based region growing is applied to the volume, Nomura said.
For false-positive reduction, differences in several feature values are used to distinguish juxtapleural from nonpleural nodules. These include surface exposure ratio (SER) RSE and surface area-to-volume ratios (SVR) RSV values. For example, the RSV values of false-positive detections have been found to be much smaller than those of true nodules, and the RSV(i) nodules (i representing the number of voxels in the surface area of the ith nodule) are smaller than those of false positives at the thin-vessel bifurcation, the authors explained in an abstract.
As a result, these feature space values are useful for removing false-positive detections in regions of vessel bifurcation, Nomura said.
![]() |
Multiple different feature values are used to distinguish nodules from false positives at the region of vessel bifurcation. All images courtesy of Yukihiro Nomura. |
![]() |
![]() |
"In the proposed method, the nodule candidates are divided into two subsets, a juxtapleural subset and a nonpleural subset," based on the number of intersection voxels of the lung volume and the nodule candidate, Nomura said. Then false-positive reduction is performed for each subset using the Mahalanobis distance-based classifier.
![]() |
False-positive reduction is based on dual classifiers, a juxtapleural subset based on the number of intersections of the lung volume and the candidate lesion, and the nonpleural subset for other values. Then the two subsets are processed by the classifier. |
![]() |
The use of dual classifiers (above) was able to distinguish juxtapleural from nonpleural nodules. fROC curve (below) showed a reduction from 15.4 to 8.1 false positives per case with the use of a dual classifier. |
![]() |
"At 90% sensitivity, the number of false positives was reduced from 15.4 to 8.1 by changing the single classifier to dual. There were also newly detected juxtapleural nodules with use of the dual-classifier scheme," Nomura said.
The preliminary results show that using dual classifiers related to juxtapleural and nonpleural nodules can potentially improve the performance of lung CAD algorithms, he said.
![]() |
New nodules were detected using the dual classifier that were not detected using the single classifier. |
Nomura and colleagues' future plans include improvement of the classification into two subsets using large datasets, and introduction of new features.
By Eric Barnes
AuntMinnie.com staff writer
November 10, 2008
Related Reading
CAD detects more lung nodules but not necessarily more cancer, September 24, 2008
CAD steals show from U.S. research database, August 12, 2008
CT databases aimed at lung imaging research, August 8, 2008
CAD helps residents identify lung nodules on DR, May 20, 2008
Criteria determine CAD mark sensitivity, March 20, 2008
Experience may make a difference with lung CAD, February 4, 2008
Copyright © 2008 AuntMinnie.com