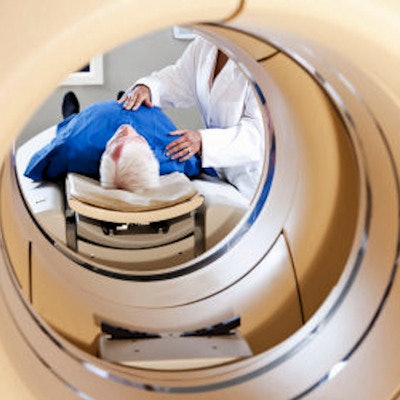
An artificial intelligence (AI) algorithm shows a promising level of accuracy for identifying normal and abnormal results on FDG-PET/CT exams, and it could potentially help radiologists avoid missed findings, according to research published online October 28 in the Journal of Digital Imaging.
A team of researchers led by Dr. Tomomi Nobashi of Stanford University trained nine different convolutional neural networks (CNNs) to classify FDG-PET scans as either normal or abnormal. In testing, an ensemble model that combined multiple CNNs yielded the best results, achieving 82% accuracy.
"If prospectively validated with a larger cohort of patients, similar models could provide decision support in a clinical setting," the authors wrote.
Normal gray matter has a high-background glucose metabolism on brain FDG-PET exams, resulting in a low signal-to-background ratio. This potentially increases the possibility of missing important findings in patients who have intracranial malignancies, according to the researchers.
"This limitation could conceivably be mitigated with the aid of a deep-learning classifier that can distinguish normal versus abnormal FDG activity in brain PET images, augmenting the interpretation of FDG-PET/CT studies by radiologists and nuclear medicine specialists," the authors wrote.
To investigate further, the researchers trained and evaluated nine 2D CNNs to detect abnormalities of brain glucose metabolism in cancer patients. Although 3D CNNs can take advantage of the entire volumetric data while leveraging the context from adjacent slices, these models are computationally intensive, require expensive hardware, and are prone to overfitting, according to the team.
On the other hand, 2D CNNs make predictions on a slice-by-slice basis and don't exploit the actual voxel-wise rich data available in 3D images such as FDG-PET/CT studies.
"With the goal of overcoming this disadvantage of 2D CNNs, we hypothesized that 2D-CNN models with multiple axes (axial, coronal, and sagittal planes), multiple window intensity levels, and their ensembles could accurately classify FDG-PET images of the brain on scans of cancer patients as normal or abnormal," they wrote.
They trained the algorithms using 100 normal and 100 abnormal FDG-PET scans acquired on four different scanners from GE Healthcare. Testing was performed on 89 exams, including 50 normal and 39 abnormal studies.
The researchers found that each axis of the individual models had a different optimal window setting for classifying normal and abnormal scans. An overall model that averaged the individual probabilities of all the individual models yielded the best performance.
Performance of CNN ensemble model for characterizing FDG-PET scans | |||
Sensitivity | Specificity | Accuracy | |
Overall ensemble model | 69.2% | 92% | 82% |
"2D CNNs accurately classified normal and abnormal brain FDG-PET scans," the authors wrote. "Particularly, ensemble models with different window settings and axes showed improved performance over individual models."
The researchers noted that 2D-CNN data augmentation methods are complicated and, if larger training datasets are available, can probably be avoided by using a 3D CNN.
"However, given the lack of a larger dataset, our results suggest 3D medical images such as PET scans can accurately be classified with 2D CNN using ensembled models," they concluded.